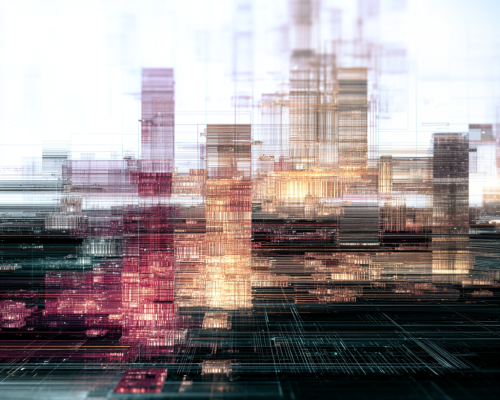
From Hype to Reality - Fighting Fraud with Synthetic Data
20.05.2025
When ABCBank unveiled its slick “onboarding in minutes” campaign, the C-suite toasted a surge of sign-ups, but Omar, the fraud lead, wasn’t celebrating. That influx hid a darker truth: bots, mule operators, and identity farms attempting to churn out fake profiles by the thousands. Omar’s team tightened rules - blocking duplicate-device enrollments and geolocated sign-ups outside the country - but they still couldn’t simulate every crafty evasion tactic.
How could he stay ahead of synthetic identities, mule accounts, and VPN-masked applications? The answer: build test cases for all combinations of critical signals. Sounds daunting - unless you generate those scenarios on demand with synthetic data.
Synthetic data has been around for decades, so they are not something novel. However, the term became more common as a result of the focus on data privacy (e.g., GDPR and PII) and even more with modern AI/ML algorithms, which require vast amounts of data (particularly image models).
Synthetic data is artificially generated information that emulates the statistical properties, structure, and patterns of real-world data without containing any actual records. It is produced through algorithmic processes, such as generative adversarial networks, agent-based models, or statistical simulators, to provide realistic, privacy-safe datasets.
Synthetic data is born in code, not captured from customers. Rather than parsing through sensitive transactions or waiting for rare edge cases to occur, we can generate transactions, device fingerprints, user journeys, or customer profiles that look and behave like the real thing. Think of it as a sandbox for your anti-fraud engines - build any scenario, bad or worse, then test how your defenses hold.
Why should fraud professionals care?
Though we will focus on the fraud domain in this article, there are many use cases where synthetic data is extremely valuable, if not mandatory (e.g., LLM, autonomous vehicles, computer vision, robotics and control systems, AR/VR prototyping, medical imaging & diagnostics). Even companies like Nvidia are huge proponents of synthetic data and its use across various verticals and a variety of purposes [1].
The main benefits:
Boosting model training – Crafting rare scenarios like credential-stuffing bursts and impossible geo-hops to accelerate model tuning, researchers at J.P.Morgan generated matching synthetic samples. Feeding these into their fraud model allowed them to retrain on those rare patterns before they ever hit production, resulting in a significant jump in detection rates [2].
Balancing data classes - Real fraud is much rarer than normal behavior; models often miss some bad cases. With synthetic data, we can create equal numbers of fraud and non-fraud examples, which often improves the accuracy of the model [3],[4].
Bias reduction - The Model built on data from one region may misfire when rolled out globally. Instead of fighting imbalanced real data, teams can inject synthetic profiles across geographies, age groups, and device types to smooth out hidden biases before deployment and fill in the potential underrepresented classes or patterns [5].
Testing rules - A bank trying to defeat synthetic identity fraud (criminals faking social profiles to dodge KYC) can generate plausible Name + DOB + SSN combinations and pair them with device data. This approach will allow them to fine-tune their rule thresholds with confidence.
Secure vendor collaboration - AI providers need data to train models, but giving access to production-level data (even anonymized) isn't without risk. Synthetic data allows vendors to train models that behave just like production-level data, without any real-world risk (e.g., model performance comparison in [6]).
Generating synthetic data that truly mirrors real-world information isn’t straightforward - it usually demands deep domain knowledge and the right tools to capture the same statistical patterns, formats, and quirks.
At the same time, forecasting future scenarios or rare events means carefully examining all related datasets - something smart algorithms can manage more effectively and at scale.
To get it right:
Keeping these guardrails in place ensures your synthetic sandbox remains a faithful, production-grade test environment.
Ready to press play on an endless stream of fraud scenarios?
References:
[1] Nvidia
[2] J.P.Morgan
[3] clearbox.ai
[4] medium.com
[5] ydata.ai
[6] medium.com